Automotive User eXperiences Research
A major component of the U.S. Department of Transportation’s (DOT) mission is to focus on pedestrian populations and how to enable safe and efficient mobility for vulnerable road users. However, evidence states that college students have the highest rate of pedestrian accidents. Due to the excessive use of personal listening devices (PLDs), vulnerable road users have begun subjecting themselves to reduced levels of achievable situation awareness resulting in risky street crossings. The ability to be aware of one’s environment is critical during task performance; however, the desire to be self-entertained should not interfere or reduce one’s ability to be situationally aware. The current research seeks to investigate the effects of acoustic situation awareness and the use of PLDs on pedestrian safety by allowing pedestrians to make “safe” vs. “unsafe” street crossing within a simulated virtual environment. The outcomes of the current research will (1) provide information about on-campus vehicle and pedestrian behaviors, (2) provide evidence about the effects of reduced acoustic situation awareness due to the use of personal listening devices, and (3) provide evidence for the utilization of vehicle-to-pedestrian alert systems. This project is conducted in collaboration with Dr. Rafael Patrick (ISE), supported by the Center for Advanced Transportation Mobility and ICAT.
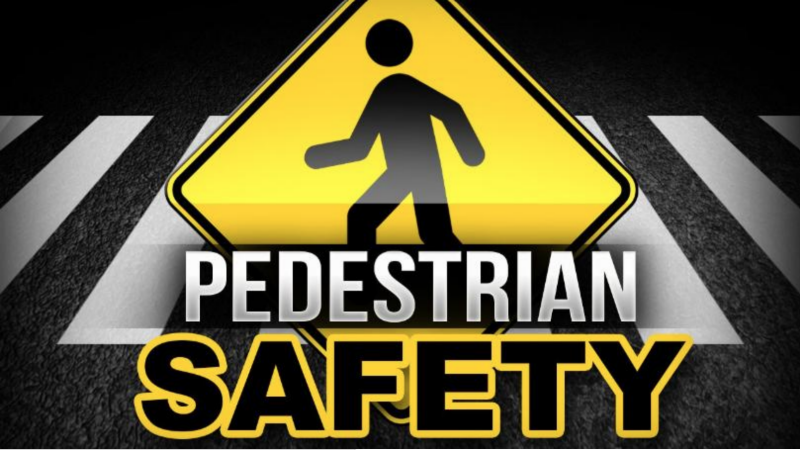
Automakers have announced that they will produce fully automated vehicles in near future. However, it is hard to know when we can use the fully automated vehicles in our daily lives. What would be the infotainment in the fully automated vehicles? Are we going to have a steering wheel and pedals? To make a blueprint of the futuristic infotainment system and user experience in the fully automated vehicles, we investigate user interface design trends in industry and research in academia. We also explore user needs from young drivers and domain experts. With some use cases and scenarios, we will suggest new design directions for futuristic infotainment and user experience in the fully automated vehicles. This project is supported by our industry partner.
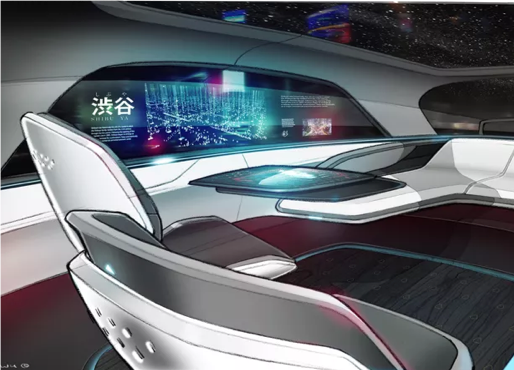
Automated Vehicles (AVs) are the next revolution in private surface transportation solutions. An SAE Level 5 vehicle allows passengers to completely disengage from driving, and instead remain engaged in a Non-Driving Related Tasks. The combination of such activities has been known to make passengers feel motion sick. Despite the advantages of highly automated vehicles, motion sickness in passengers is an important human factors challenge. This project seeks to explore the inducers of motion sickness in such conditions, apply them to artificially induce motion sickness in a controlled environment, and investigate methods of mitigating motion sickness in passengers of automated vehicles through the use novel anticipatory auditory displays. A portion of this work has been supported by the Perception and Performance Technical Group of the Human Factors and Ergonomics Society.
Milo and NAO robots are used as an embodied intelligent agent.
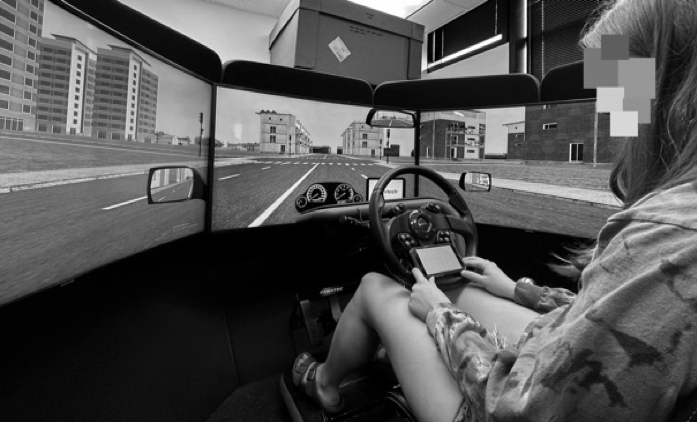
In addition to the traditional collision warning sounds and voice prompts for personal navigation devices, we are devising more dynamic in-vehicle sonic interactions in automated vehicles. For example, we design real-time sonification based on driving performance data (i.e., driving as instrument playing). To this end, we make mappings between driving performance data (speed, lane deviation, torque, steering wheel angle, pedal pressure, crash, etc.) with musical parameters. In addition, we identify situations in which sound can play a critical role to provide better user experience in automated vehicles (e.g., safety, trust, usability, situation awareness, or novel presence). This project is supported by our industry partner.
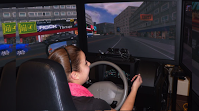
This project investigates human factors issues in automated vehicles, particularly the potential vulnerabilities of sensors to hacker attacks. As AVs rely heavily on sensor data for real-time decision-making, this project aims to understand how these cybersecurity threats can impact human safety and trust in AV systems, and what strategies can be implemented to mitigate these risks.
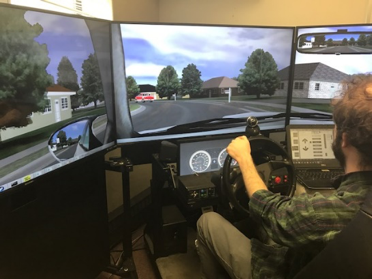
Up to 67% of accidents at Highway-Rail Grade Crossings are due to motorists not stopping in time. As part of ongoing efforts to improve driver behavior at rail crossings, and improve safety at active and passive crossings, this projects investigates the design of auditory and visual in-vehicle alerts. Furthermore, driving simulator studies incorporating different crossing scenarios and crossing types are conducted to test for the effectiveness of multimodal in-vehicle alerts. This project is a collaborative project with Michigan Technological University, and is funded by the Department of Transportation’s Federal Railroad Administration. Future work will include conducting real-world studies through refined alerts with prototypes for intelligent warning systems to reduce the likelihood of vehicle-train accidents at highway-rail grade crossings.
* This project has been supported by US Department of Transportation (DOT) / Federal Railroad Administration (FRA).
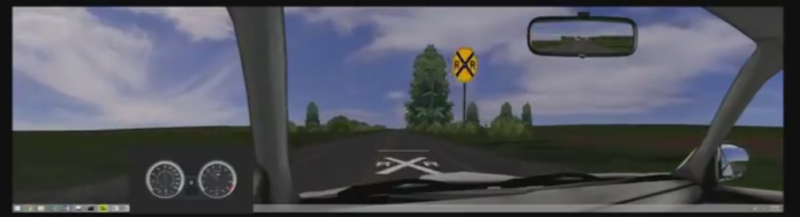
This project investigates train operators' mental workload, fatigue, and situational awareness, especially during periods of multitasking or low-engagement scenarios. The goal is to assess how these factors impact operational safety and develop strategies to mitigate risks associated with reduced attention and cognitive strain during extended periods of monitoring.
* This project has been supported by US DOT (Department of Transportation), and FRA (Federal Railroad Administration) in collaboration with AtkinsRéalis.
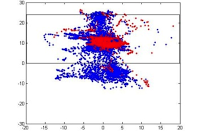
** image credit : https://railroads.dot.gov/sites/fra.dot.gov/files/fra_net/2940/TR_Cognitive_Collaborative_Demands_Freight_Conductor_Activities_edited_FINAL_10_9_12.pdf
This study investigated the impact of in-vehicle agents’ voice gender (female vs. male) and embodiment (embodied robot agent vs. voice-only agent) on user perception and eye-tracking data. The study's outcome contributes to the design of in-vehicle agents in automated vehicles by providing unconventional results of the female voice being perceived as more likeable and competent, whereas the male voice improves perceived safety. The Northrop Grumman Undergraduate Research Award sponsored this study.
* This study has been supported by the Northrop Grumman Undergraduate Research Funding.
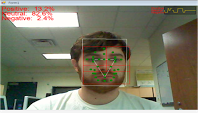
In-vehicle touchscreen displays offer many benefits, but they can also distract drivers. We are exploring the potential of gesture control systems to support or replace potential dangerous touchscreen interactions. We do this by replacing information which we usually acquire visually with auditory displays that are both functional and beautiful. In collaboration with our industry partner, our goal is to create an intuitive, usable interface that improves driver safety and enhances the driver experience. This project was supported by Hyundai Motor Company.
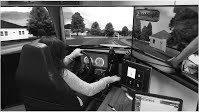
This research investigates how happy and sad music affects the driving behavior of individuals in different emotional states. Using a high-fidelity driving simulator and physiological monitoring, we examine drivers' responses to emotional music, especially happy and sad stimuli. The study aims to provide insights into the interaction between music, emotions, and driving performance, contributing to safety on the road. Conducted in the Mind Music Machine Lab at Virginia Tech under Dr. Myounghoon Jeon, this project explores innovative approaches for road safety through understanding the emotional dynamics of driving. The research team includes (Joanna) Ziming Fang and Dayna Rohmann, who are actively working on this project.
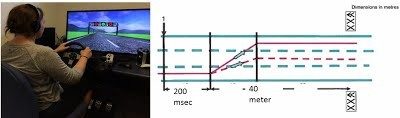
This research series explores the potential of advanced in-vehicle intelligent agents (IVIAs) to enhance driver-vehicle interaction, focusing on emotional aspects and user adaptation. Our studies investigate how empathic responses from IVIAs can mitigate negative emotional states and improve driving performance across various scenarios. We examine the effects of different empathy styles (cognitive vs. affective) on drivers' emotional states and driving behaviors.
Additionally, we explore how accent variations (e.g., Standard American vs. Southern US) influence drivers from diverse linguistic backgrounds (non-Southern US, Southern US, and international drivers). We analyze changes in subjective evaluations, trust levels, situation awareness, and driving behavior when interacting with these adaptive IVIAs.
Through driving simulator studies and empirical data collection, we aim to develop comprehensive design guidelines for empathic and user-adaptive in-vehicle agents. Our ultimate goal is to contribute to the creation of more personalized, safe, and effective driving experiences in future mobility systems, accommodating various levels of driving automation and user preferences.
* This prjoect has been supported by the VT Destination Area 2.0 Fund.
In this project, we have been developing computational models based on quantitative measures of human driver behaviors, such as eye gaze patterns and response times when they receive various types of information, including visual and auditory feedback, takeover displays, or cyber attacks while driving. By applying computational modeling techniques, including Bayesian methods, Hidden Markov Modeling, and Queing Network-Model Human Processor (QN-MHP), we aim to optimize human behavior predictions, providing insights into how drivers interact with automotive systems and refining these models to enhance safety and performance in mixed-driving environments.
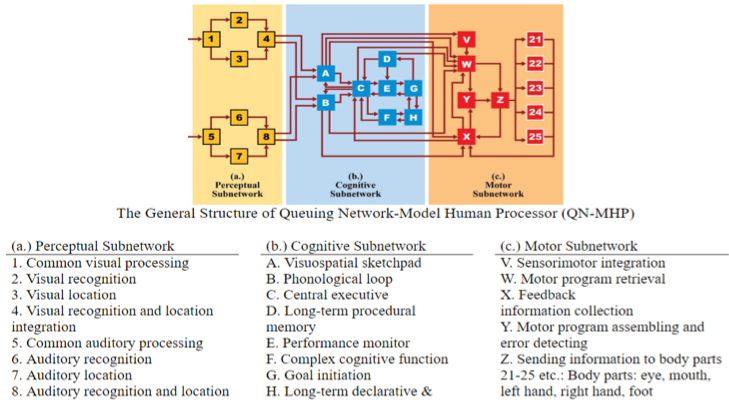
Sponsored by the Northrop Grumman Undergraduate Research Award, this project utilizes assistive robots in a driving simulator to study the effects of reliability and transparency of the in-vehicle agents on trust, driving performance, and user preference. The objective of this on-going research is to determine the best level of transparency in an AI agent to optimize driver situation awareness, increase trust in automation, and secure safe driving behavior.
* This study has been supported by the Northrop Grumman Undergraduate Research Funding.
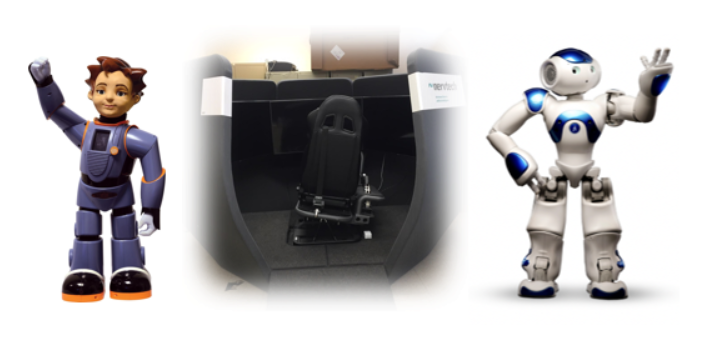
The advancement of Conditionally Automated Vehicles (CAVs) requires research into critical factors to achieve an optimal interaction between drivers and vehicles. The present study investigated the impact of driver emotions and in-vehicle agent (IVA) reliability on drivers’ perceptions, trust, perceived workload, situation awareness (SA), and driving performance toward a Level 3 automated vehicle system. Two humanoid robots acted as in-vehicle intelligent agents to guide and communicate with the drivers during the experiment. Our results indicated that trust depends on driver emotional states interacting with the system's reliability, which suggested future research and design should consider the impact of driver emotions and system reliability on automated vehicles.
* This study has been supported by the Northrop Grumman Undergraduate Research Funding.
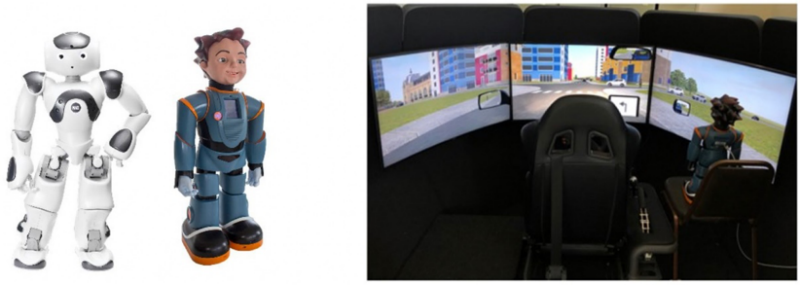